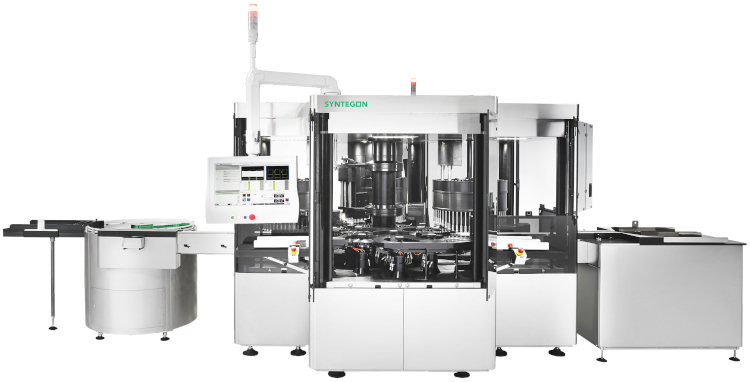
Leading biotech drug-maker and marketer Amgen recently achieved an industry first: The first fully validated visual inspection system using artificial intelligence (AI), according to Amgen’s partner for the project, Syntegon Technology (formerly Bosch Packaging Technology).
The project involved an AI retrofit to a Syntegon syringe inspection machine installed in 2015 at Amgen’s Juncos, Puerto Rico, production facility. The machine has 13 inspection stations, each performing a specific inspection task on syringes and their contents. The challenge is that it’s difficult to ensure accurate detection of unacceptable products without rejecting too many acceptable products.
“Most inspection machines are very effective at detecting defects, but they also provide a lot of false rejections that represent a significant cost to operations,” says Manuel Soto, senior principal process development scientist with Amgen’s Core Technology Team. Typically, a sort of balancing act is required in which companies must tolerate high reject counts to guarantee product safety and quality.
Amgen met this challenge using AI — specifically, deep learning technology — to address a particularly challenging problem at one critical inspection station that challenges companies industry-wide: bubbles.
How AI beats bubble trouble.
Specifically, the problem with air bubbles, especially in highly viscous parenteral (injectable) solutions, is that they can’t be easily or quickly eliminated or differentiated from unwanted particles that represent product defects. This is especially challenging when bubbles form around the syringe’s rubber stopper and can’t be sufficiently spun-away or otherwise eliminated. This makes it hard for conventional vision systems to identify the anomaly.
The results were surprising and remarkable: The particle detection rate for this critical station increased by around 70%, while the false detection rate was reduced by about 60%.
What makes the AI so much better? It’s in the software. Traditional inspection uses rule-based techniques “to perform pre-determined operations on specific areas within the target image,” explains Dr. José Zanardi, responsible for vision inspection development and applications at Syntegon. In contrast, the deep learning application uses neural networks to process images in a manner more akin to our human eyes and brain: “It looks into differential patterns and makes decisions on the ‘knowledge’ of pattern changes stored in the trained neural network.”
To get a traditional vision system to effectively differentiate between the smooth surface of a bubble and the rugged surface of a glass shard “would require an enormous amount of different settings and operations broken down to very small areas in the region of interest,” Zanardi adds.
Amgen was able to retain its machine’s preexisting optics and camera (specifically, a CMOS line-scan camera), essentially updating only PCs and software. This included a new, more powerful runtime vision PC at the upgraded vision station; and an update to the MVTec Halcon vision/deep-learning software plant engineers were already using, offline, to train the deep-learning model.
The latest news from the Syntegon side of the partnership, Zanardi says, is a full AI inspection solution “developed completely in-house,” says Zanardi, presently offered for new machines or retrofits.
Achieving validation for the AI.
The project uses a supervised implementation of deep learning that gives the administrator full control to change — or prevent changes as governed by change-control procedures. For instance, once a deep-learning model is validated, it’s locked, encrypted, and version controlled. The same goes for the runtime software at the machine level. And as with any pharmaceutical production equipment, the machine must demonstrate its performance during validation.
Benefits beyond the machine.
Maximizing the defect detection rate improves quality and safety for customers, in this case, Amgen’s patients. Reducing the number of false rejects cuts waste, reworks, and overall costs.
“The end result is that we are more effective detecting defects and we are also more efficient at not rejecting good product. So the benefit is at both ends — the quality side and the efficiency side of our operation — empowering Amgen to deliver quality products to every patient every time,” Soto says.
Syntegon’s Zanardi adds that the AI solution simplifies “vision recipes” to cut idle time and speed changeovers. In turn, the system reduces reliance on experienced engineers, who he says are traditionally “the only ones who can create and adjust traditional vision recipes.”